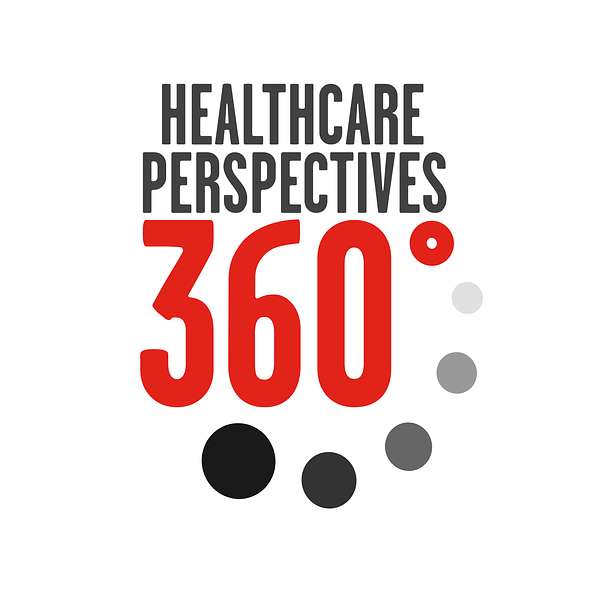
Healthcare Perspectives 360
Healthcare Perspectives 360
Danger! Recognizing the Risks of AI Use in Healthcare
This podcast explores how to recognize risks present when using AI in healthcare settings.
During this episode you will:
- Hear how AI can “hallucinate” (or make up) information
- Listen to a discussion about the potential for medical misinformation from AI
- Learn the importance of countering patients who may have been misled by AI misinformation
- Hear how some AI data sets may exacerbate care disparities for underserved populations
Link to Transcript
Coverys companies are a leading provider of medical professional liability insurance for medical practitioners and health systems. Coverys provides a full range of healthcare liability insurance options, advanced risk analytics, and best-in-class risk mitigation and education resources to help clients anticipate, identify, and manage risk to reduce errors and improve outcomes.
Med-IQ, a Coverys company, is a leading provider of clinical and risk management education, consulting services, and quality improvement solutions, empowering individuals at every level of the healthcare delivery system with the knowledge they need to continuously improve provider performance and patient outcomes.
The information provided through this activity is for educational purposes only. It is not intended and should not be construed as legal or medical advice. Opinions of the panelists are their own and do not reflect the opinions of Coverys or Med-IQ.
Music and lyrics: Nancy Burger and Scott Weber
[music]
Geri Amori, PhD, ARM, DFASHRM, CPHRM: Hello, everyone, and welcome to Healthcare Perspectives 360, a podcast dedicated to exploring contemporary healthcare issues from multiple perspectives. I'm Geri Amori, and today I am joined by Irene Dankwa-Mullan, MD, MPH, a physician executive, researcher, and thought leader in health technology innovation, currently adjunct professor at George Washington University's Milken Institute School of Public Health, Chief Health Officer at Marti Health, with expertise in the ethical use of AI in healthcare, aiming to bridge gaps in access for underserved communities and ensure personalized, precision care for equitable outcomes.
I'm also joined by Danielle Bitterman, MD, assistant professor at Harvard Medical School, and she is a radiation oncologist at Dana Farber and Mass General Brigham who has unique expertise in AI applications for cancer and AI oversight for healthcare.
Chad Brouillard, Esq., a medical malpractice defense attorney and a partner at the law firm of Foster & Eldridge LLP, in Massachusetts, with expertise in electronic health record liability, AI implications, and all things technologically related to healthcare liability and risk. Welcome to our panelists and our audience.
Today, we're talking about recognizing the risks present when using AI in healthcare. Recently, I was at a conference where I learned more about the built-in bias in large language models in AI. What I heard was that every time a search or action takes place using a large language model AI search, that search itself becomes part of the future searches. And the correctness or incorrectness of what is generated then becomes part of what is later incorporated. I was also surprised when I realized that much of the peer-reviewed research that contributes to evidence-based medicine is accessed only behind subscriptions and library locked doors. This means that an AI search may or may not have access to that information when developing its material. Also, even if it does have access to this information, we all know that evidence-based medicine is a moving target, and the evidence is constantly changing. Okay, just want to put it out there – as a consumer I'm a bit scared.
So today, we’re going to ask our experts what they see as the risks. Danielle, I'd like to start with you. We've been hearing about large language models and bias or what some people call AI hallucinations. We know it's something that's hard to explain, and it's hard to defend something you can't explain. Kind of like a black box. What are your thoughts about this?
Danielle Bitterman, MD: Yeah, that's a great question. There’s a lot of interest in AI opening the black box of large language models in AI more broadly. And what’s challenging is that the models that tend to be the highest performing models are “deep learning models” – large language models are a type of deep learning model – and those are the ones that are the more black box models opposed to the more traditional models where you can totally understand how they arrived at a decision, but they tend to not always perform as well, in some cases.
There's two sides to the black box. There's interpretability of a model, and there's explainability. And so, taking the analogy of a car, interpretability means you can say this is exactly what parts of that model change when it was making a decision. So interpretability is like if you have a car, you want your car mechanic to know how your car works. Explainability is whether the model can tell you how it arrived at its decision. So you may not need to know the details of a car mechanic to be able to drive a car safely, but you want your car to alert you when you it needs an oil change.
I think there are really important components of trust in an AI system clinicians and patients report wanting, especially to have explainability and able to feel trust in what they're doing. I think there's other ways to arrive at trust. There's generating an evidence-based showing that even if you don't totally understand how the model’s internals work, you still know that when people use these models it leads to better outcomes. So I think there's different ways at gaining evidence and gaining trust of which kind of opening the black box, providing interpretability and explainability to models can help but are not the complete solution.
Amori: Oh! Okay! That feels like something a little over my head. Hey, Irene – and I want to be able to understand things, too – Irene, we know that underserved populations are unequally represented in that data from the libraries or the things we can get that go into the large language models. And they are frequently underrepresented in the research considered for the norming of best practices or even the development of medications. So what do you see as the dangers there?
Irene Dankwa-Mullan, MD, MPH: Yeah, thanks for that question. That's really a crucial issue. I'm really glad you brought it up because the underrepresentation of populations that are underserved in data that trains these large language models, and even more broadly, in clinical research, drug development really, really poses serious dangers. One of the huge dangers is reinforcing these biased practices. Because as you mentioned, many best practices in healthcare normed on these data or signs that really exclude marginalized populations or groups.
When systems rely on these standards without questioning their applicability in different populations, then they risk really sort of codifying these biased practices into these automated decision-making tools. And so, it creates a vicious cycle where these inequities are not only maintained but really become harder to identify and address because they’re already embedded in the technology that’s seen as objective and seen as neutral.
There's also a risk of eroding trust. These populations, especially patients with sickle cell, already have valid reasons to distrust healthcare systems. When these AI systems then provide inaccurate or biased recommendations, it further alienates these communities. But, the huge one for us clinicians is the issue of safety – the issue of inappropriate treatments, missed diagnosis. All of those directly impact in patient safety. So it's particularly troubling for populations that already face barriers to accessing timely and appropriate care.
Amori: Yeah, yeah, I can see that. So Chad, I happen to know a bunch of risk-averse people living in the risk management world as I do. And I know people that have stopped using voicemail, you know, answering machines with their own voices. They started using the standard voice because they've been hearing about how easy it is for someone to deepfake your voice and take your voice print and use it. While that may not happen to most of us normal people, and it could probably happen to famous people, it does raise the question for me about how voice prints could be used to spread disinformation or what we call a deepfake. What is the danger of deepfake to spread medical disinformation, and could the healthcare system as well as patients be fooled?
Chad Brouillard, Esq.: Sure Geri, I'm happy to answer that question. I mean, I think the greatest probability of that type of risk really focuses around public health officials or other types of public medical professionals who are disseminating information. You know, it is very easy to use tools like social media to take something like a deepfake and make it appear as if a trusted medical authority is telling you what or what not to do with your health in general. And that can be disseminated from whoever has that agenda.
I mean, we've all seen this as a society. We've been living through this for the past however many years. So it makes it very problematic. I understand, when you have patients who come in who have disinformation and basically believe it to the hilt, and it may interfere with their medical care. I mean, I think, secondarily, there is a risk. I think health care is infrastructure, and both criminal enterprises and hostile foreign countries sometimes target healthcare as infrastructure.
And so, if you can replicate a doctor's voice – and in fact, there are some legitimate AI applications that are trying to be used for things like postoperative follow-up phone calls in which they deploy kind of like a chatbot but it's with a deepfake of the doctor's voice to kind of bond the connection with the patient. But you can imagine using a technology like that to scam people, to convince them to do things that are against their health for a variety of reasons. But I don't think that's the more likely application of deepfakes. I think it's more of the public health officials and spreading misinformation or disinformation that way.
Amori: Okay. Well, now that you've convinced me I don't need to worry that nobody is ever going to want to deepfake my voice, I can go back to using voicemail. I would like to ask you, Irene, though a lot of people, not everybody, but people research stuff online before they come to see their doctor because they want to be prepared. And so, do you ever wonder about the validity of information brought in by your patients? And what are the implications of deepfakes being mistaken as true authority in healthcare?
Dankwa-Mullan: Absolutely! I mean, I'm a scientist, so I'm always looking for evidence, and I do wonder about the validity of information that patients bring in, especially now when misinformation and deepfakes are becoming so sophisticated. Patients today have access to an overwhelming amount of information online, and not all of it, as we say, is reliable. And so, when a patient comes in referencing a treatment that they saw on social media or a so-called expert they watched on YouTube, it can be challenging really to untangle what's credible from what's misleading.
And the implications are really, really concerning. I mean, imagine a scenario where a deepfake video of a well-known physician or a public health authority, as Chad was saying, is circulated promoting a harmful or unproven treatment. And many people might take the information at face value assuming it’s legitimate, especially when it comes across as being authoritative and well produced. Patient safety is at risk. If patients act on false information, the consequences could be severe like misdiagnosis, inappropriate treatment, even refusal of the necessary care are all real dangers. So it's a challenging situation.
And healthcare relies heavily on trust between patients and clinicians or healthcare providers. And so, if patients start to question the authenticity of what they see, what they hear from their doctors or healthcare professionals because they’re aware that deepfakes exist, it could make it even harder for clinicians to build and maintain that trust. And patients might start second-guessing legitimate information, which further complicates care delivery and adherence to treatment plans.
Amori: Yeah, yeah. I could see that. Danielle, you’re an educator. You teach in medical school as well as practice. So do you think there's a difference in the risk exposure for the use of AI as a practitioner versus the use of AI in medical education?
Bitterman: The use of AI in healthcare will start earlier but more broadly because of the perceived differences in risks, which I think is reasonable, but I actually think the type of risk that we care about from AI in medicine versus AI in medical education is not different. We care about the ultimate harm to patients. What is different is the time and the immediacy of the impact of the risks of the two use cases and the durability of impact. They are actually kind of inversely related.
So using AI in medicine has a much more immediate risk. So if you use it to care for patients and it hurts a patient that’s an immediate risk. Whereas the use of the AI in medical education, as you proceed to training, you start with lots of oversight from other clinicians and gradually you become more and more independent. So that kind of risk if you don’t quite understand something or because something went wrong in your education is mediated for a long time. There’s a lot of points where a human can come and say, oh, no, your understanding is wrong. So the immediacy of impact is less there.
However, the durability of impact with AI in medical education is actually, I believe, greater than that durability of impact of using AI in healthcare because if we have cohorts of trainees who have used AI as part of their medical education and that starts to compromise their skills, if it does, that's going to be hard to reverse because you start losing generations of people who might have been there to identify errors and things that have gone on in training. So it's potentially more impactful but just over a longer period of time.
But luckily because of that difference in the speed of which you can hurt a patient I believe there’ll be time to kind of see, okay something is going wrong, we have to go back and change our education approaches. That said, there's also a risk in not using AI that is likely to support medical education.
Amori: Yep, I see it. Everything has a ying and a yang, doesn’t it? Both sides. The good and the bad. So, Chad, I know you are all about patient rights and responsibility and protection and all of that, so what are your thoughts about the use of large datasets of patient information to train the AI processes like feeding it a huge bunch of radiology to help it learn about cancers, for example? What are your thoughts about the risks and benefits of that?
Brouillard: Well, I mean, I think first I would accentuate obviously the benefits of doing that, right? I think that if you're going to have an AI application, you're trying to eliminate bias, and you're trying to get the largest dataset that you can to work with to get a better product. So I think, on one hand, it's kind of a necessary part. What we're trying to do clinically with AI is to train it better, and we need that data on one hand. But on the other hand, patients have rights, too. There's a whole long history about being able to use patient information for research purposes. Obviously that's embedded in the HIPAA privacy rules, and I know medical systems take great care when they're vetting research projects. It's a very sort of complicated process.
Generally, we do have to keep in mind that with AI sometimes doing things that were completely appropriate a decade ago, just taking the patient's name off of a record may not be definitive in terms of de-identifying medical information these days. Very often you can take what's left in the medical record and run it through an AI that’s looking at social media and other internet-reported information about a patient, and it's very easy to reconstruct who that patient is. So it makes the whole process, I think, a little bit more challenging in this day and age.
And patients certainly feel like they should have some say in whether their information is being used to train AI. And the different products do things differently about how they handle that data, whether it's being stored locally somewhere, whether it's being hosted somewhere in the cloud, whether they allow other software pieces to use that medical information. Whenever a healthcare organization is considering using an AI software vendor, they have to have really complicated discussions about the use of patient data, the security of patient data, making sure they have things like business associate agreements in place to make sure that their patients’ data is being safeguarded.
Amori: So that makes it complicated. We want to give the AI all the information we can so that it has the biggest database to pull from, and yet, we need to be able to protect patients as well. And so, it's always that balancing act is what I'm hearing you say.
Brouillard: Right.
Amori: Yeah. We’re running short of time here, so I'd like to go to our last question of the day, which is my favorite question. For each of you, given the dangers of AI in healthcare, what is the one thing you would like our audience to walk away with today? The one thing you want them to remember from today's discussion. And Danielle, I'd like to start with you.
Bitterman: The one thing that I would want people to take away is right now what's causing a lot of the challenges with risk mitigation in healthcare AI is how rapidly the technologies are advancing. They really are advancing faster than we can do what we would normally want to do – large-scale clinical trials to demonstrate benefit or even real implementation studies for lower-risk application. New models are coming out weekly, and we can't keep up with our traditional ways of evaluating.
We need as a community to find new, still safe, ways to rigorously evaluate technologies that are evolving so rapidly. So, ensure that we're getting real innovations to patients but not exposing to too much risk. So, don't have a solution for that, but that, to me, is the key challenge right now with risk mitigation.
Amori: Okay, that's a good one. That's something we need to address. Irene, what about you?
Dankwa-Mullan: So patients really want to trust the information they receive from AI and make sure that it's accurate, it's unbiased, it’s reflective of their unique needs and backgrounds. And so, with the growing threats of deepfakes, misinformation, building trust is more important than ever. One thing I'd want to mention, which is also related, is traditional medical ethics always emphasize patient autonomy. You want to ensure that patients can make informed decisions about their care. And so, this is where it comes in – AI in healthcare introduces these opaque decision-making processes. Making sure that information is as accurate as possible. And hopefully, we're working – the entire community, patients, healthcare providers, our legal partners – are all helping to ensure that there are processes in place for that.
Amori: That’s an important point about the patients being able to trust. Chad, what will be the one thing you would like us to take away today?
Brouillard: From today's discussion, I think I’d really like to focus on the patients’ right to the protection of their own information. I think that's very difficult in a fast moving AI market, but I think it is very much an obligation on behalf of the institutions and providers that are implementing these tools clinically to have really robust discussions up front about what is happening with their patients’ data, how it's being used, is there a possibility that it's going to be shared or disseminated either through the program itself or based on other things that the company is working on and making sure that legal protections in the forms of business associate agreements are in place.
Amori: Excellent. Excellent. Well, thank you, everyone. This has been a very enlightening conversation and an interesting conversation. I want to thank our panelists. And I want to thank our listeners for being here with us today. And I look forward to seeing all of you again next time we look at a healthcare issue from a Perspective 360.
[music]