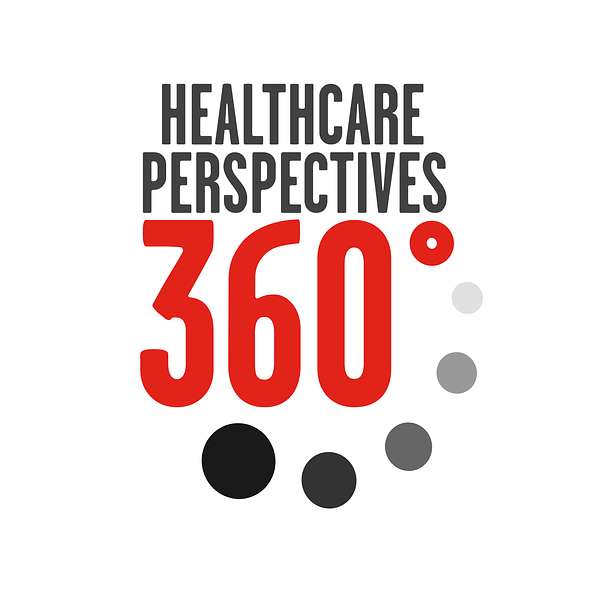
Healthcare Perspectives 360
Healthcare Perspectives 360
The Explosion of AI in Healthcare
This podcast explores the proliferation of artificial intelligence (AI) use in healthcare.
During this episode you will:
- Hear how AI has rapidly evolved for use in healthcare and how it is currently being used in healthcare
- Understand the different types of AI that are used in healthcare in a broad sense
- Hear the importance of reliable data sets for AI “learning”
Link to Transcript
Coverys companies are a leading provider of medical professional liability insurance for medical practitioners and health systems. Coverys provides a full range of healthcare liability insurance options, advanced risk analytics, and best-in-class risk mitigation and education resources to help clients anticipate, identify, and manage risk to reduce errors and improve outcomes.
Med-IQ, a Coverys company, is a leading provider of clinical and risk management education, consulting services, and quality improvement solutions, empowering individuals at every level of the healthcare delivery system with the knowledge they need to continuously improve provider performance and patient outcomes.
The information provided through this activity is for educational purposes only. It is not intended and should not be construed as legal or medical advice. Opinions of the panelists are their own and do not reflect the opinions of Coverys or Med-IQ.
Music and lyrics: Nancy Burger and Scott Weber
[music]
Geri Amori, PhD, ARM, DFASHRM, CPHRM: Hello, everyone, and welcome to Healthcare Perspectives 360, a broadcast dedicated to exploring contemporary healthcare issues from multiple perspectives. I'm Geri Amori, and today I'm joined by Irene Dankwa-Mullan, MD, MPH, Chief Health Officer at Marti Health, a physician executive researcher and thought leader in health technology innovation, currently adjunct professor at George Washington University's Milken Institute School of Public Health, Chief Health Officer at Marti Health, with expertise on the ethical use of AI in healthcare, aiming to bridge gaps in access for underserved communities and ensure personalized, precision care for equitable outcomes.
I'm also joined by Danielle Bitterman, MD, assistant professor at Harvard Medical School, and she is a radiation oncologist at Dana Farber and Mass General Brigham who has unique expertise in AI applications for cancer and AI oversight for healthcare.
And finally, Chad Brouillard, Esq., a medical malpractice defense attorney and a partner at the law firm of Foster & Eldridge LLP in Massachusetts, with expertise in electronic health record liability, AI implications, and all things technologically related to healthcare liability and risk. Welcome to our panelists, and welcome to our audience.
Today, we're going to talk about the explosion of artificial intelligence, also known as AI, in healthcare. I'm going to take a moment first to give us a context for the next series of 4 podcasts on AI. AI was initially described in the 1950s—which is, what, 75 years ago—as expert computer systems that could mimic human intelligence. We have to remember that in the 1950s, computers were these giant machines that took up an entire room. So in 1950, when Alan Turing published his iconic book Computing Machinery and Intelligence, and he introduced the Turing Test, which was a method for measuring a machine's intelligence, most people felt they would never have to deal with the ball being set into motion.
In 1952, Arthur Samuel created a program that could learn to play chess independently, which was fun and interesting. But it wasn't until 1955 that John McCarthy coined the term “artificial intelligence.” In the early 2000s, machine learning was used in computer processes to address lots of problems in academia and industry, but it wasn't until 2017 that transformer architecture was used to produce what we call generative AI applications. That was the major shift in independent computer thinking.
By the 2020s, huge investment in transformer architecture led to rapid development and release of large language models like ChatGPT. But ChatGPT didn't last long as the only option. Suddenly, in 2025, AI is everywhere and doing everything. Every day, it seems, new forms of AI are being released that can do things from writing books to providing information. In our personal lives, we have Alexa, Siri, chatbots, voice-prompted search engines, Spotify giving you your year-end summary of all the music you listen to. Seemingly, it all feels personalized to you.
So this is a movement that started 75 years ago. Who knew the ways that machines would be able to mimic the logic and thought processes of humans and, in some cases, even exceed them? And for every positive advancement, there comes new risks, new areas of concern, and new considerations for safe and ethical usage.
Today, and in the following sessions, we are going to focus on the exploding use of AI in healthcare. What is happening, and what do we need to know? In other podcasts in this series, we will look at what we need to be careful about, what we should be excited about, and how to think about the ethical and practical implications for AI utilization. So, I'm going to start by asking our panelists to name some of the ways you see AI being used in healthcare. But before I begin, is it okay with our panelists if I call you by your first names?
Female: Yes.
Chad Brouillard, Esq.: Of course.
Amori: Oh, great. Thanks. Danielle, I'd love to start with you. You work in a world-renowned cancer treatment facility. What changes have you seen in the use of AI inside your facility in the last 4 to 5 years? Are there more robots to run errands and bring medications and supplies? Just what is happening?
Danielle Bitterman, MD: This is a great question. I think it's a perfect place to start because we really are at an inflection point and AI taking off in healthcare and really starting to make its way into the clinic, beyond the robots that we see delivering medications in the hallways. I'll start with my role as a radiation oncologist.
Radiation oncology is a pretty technical field. It’s one of the earlier fields to start actually being touched by artificial intelligence. And we have been interacting with artificial intelligence methods increasingly over the past, I would say, certainly 5 years. But especially over the past 2 to 3 years, where AI methods are increasingly being used to help improve the efficiency with which we develop our radiotherapy plans, which are the plans we use to create safe, conformal treatments for our cancer patients. We're increasingly seeing methods that assist physicians and assist others in the clinic to help get develop more targeted plans more efficiently, which allows us to deliver our treatment in a more personalized and adapted fashion in ways that we never were before.
From the large language model side, which is separate from what we're currently using for AI-based radiotherapy planning, we are now starting to see integration of large language models natively within our electronic health record systems, primarily to support administrative processes in terms of assisting physicians with writing notes, with ambient documentation, assisting physicians, and responding to patient messages, which primarily, hopefully, will help to adjust the clinician burnout crisis but that also secondarily serves our ultimate goal of improving care for patients. Because clinicians that are better supported are able to take better and more personalized care of our patients.
Amori: Wow. That's a lot. And the notion of helping with burnout, I think we're going to talk more about that later, but that's an interesting idea. Irene, I'd like to ask you now, I gather a lot of your work is on the outpatient side, whereas Danielle's is primarily on the inpatient side. Where is AI being used in your work? Do you use, I don't know, chatbots, or automated replies, or virtual assistants to take care of appointments or, I don't know, refill requests?
Irene Dankwa-Mullan, MD, MPH: You’re right. I mean, I do focus a lot on the outpatient side. And when it comes to managing chronic diseases like sickle cell, AI really has some promising applications, right? Marti Health, basically, is a healthcare organization focused on providing comprehensive, patient-centered care to communities that have been underserved and beginning with patients with sickle cell, right?
And so, sickle cell patients often require frequent and coordinated outpatient care to manage their crisis, pain crisis, prevent complications, and keep up with medications and all the appointments. So, AI is starting to make a difference here, both in care coordination and in making those day-to-day managements a bit easier for patients and families and caregivers.
So, one of the most effective uses in this space is predictive analytics. So by analyzing patterns in a patient's data, like pain crisis, lab results, even social factors, AI can predict when a patient might be at risk for a crisis or hospitalization. This allows the care teams to step in, proactively adjust treatment plans, even arrange preventive outpatient visits before those things escalate.
And so chatbots, virtual assistants are all playing a role. In fact, we've been exploring AI-powered chatbot that can handle things like appointment scheduling, medication refill request, and even symptom check-ins. Because these patients, who often have to juggle multiple medications and frequent visits, having an available 24/7 to answer questions, send reminders can really help reduce the burden.
We also have seen AI tools that help to streamline care coordination between specialists, right? So, for patients with sickle cell, it's not just about hematologist. It's often coordinating with pain management, cardiologists, even mental health providers, social workers, right? We're aiming to provide holistic care. And so, AI systems—there’s actually AI systems that can actually integrate EHR data and automate care plans, help to ensure that these patients, or everyone on the care team, has the most up-to-date information without needing to really sift through notes and labs.
But I also want to mention there's also remote monitoring, right? That wearables that can track oxygen saturation, heart rate, combined with AI to analyze data, can really help monitor these patients at home and catch early warning signs of complications.
Amori: We’re going to hear more about that later, I am sure. And I just, I mean, it makes me wonder, how did we practice medicine before? I mean, there's tools that can do so much.
Hey, Chad, as an attorney, has the presence of AI in the healthcare facility changed at all the way you approach working with healthcare organizations when you are investigating the causes of medical error or potential litigation?
Brouillard: Thank you, Geri. That's a great question. And I'm going to push back on one of your comments just before the question, which I think will help put AI in context in terms of what I do and, namely, that we've had things like chatbots. We've had algorithms that help with fields like pathology and radiology, actually, for decades at this point. I believe the first FDA-approved AI algorithm had to do with pathology, and it was approved in 1995.
Amori: Wow.
Brouillard: Although, I do agree with Danielle that we are at an inflection point because the type of AI that we're dealing with now has a tremendous ability to do things like sift through unstructured data, and it's kind of unprecedented in that sense. But unlike a lot of new medical technologies, usually, as the lawyer, I'm saying, well, wait a minute, let's take a step back and let's see how the courts deal with this because I have no idea what they're going to do with this, right?
But when it comes to AI, we actually do have somewhat of a corpus of case law that is dealing with the issues relative to the use of computer systems or algorithms, even if we call it old AI, which gives us some guidance. But, as a defense attorney who's very often working with institutional clients or individual clients, one of the chief difficulties for me is that very often the use of algorithms or the use of AI is not transparent in the medical record. And so that's very difficult when my job is telling the story of the clinician and how they delivered the care and why it was reasonable if I don't know that they actually deployed or used an AI tool which helped them come to their decision-making. So that's a very problematic thing.
But, frankly, I've dealt with it in the older form of algorithms being used and embedded, particularly in electronic health records. I had a case where, essentially, all the confidential information removed, right? But essentially it had to do with clinical decision-making and using a score which is calculated based on various risk factors. And the clinicians only had the score documented, but what was not produced in the record was the table of the algorithm actually filling in all the risk factors and then arriving at the score. So it was only through some sleuthing and working very closely with the HIM department that we were able to find out, wait a minute, this doctor…actually, it was exculpatory, right, that this doctor arrived at the score using this tool, and it was a very reasonable thing to do.
So, I'm concerned in terms of the transparency issues because even as counsel or as a clinician who's being sued 3 years after the fact might not remember that you used an AI tool. And then we have the secondary issue of if an AI tool was used, is the AI modeling being preserved? Because most AI systems are not set up to retain past models. So we kind of have retention issues as well, which can make my job a little bit thorny.
But overall, that's sort of what I would say when dealing with AI. It's something that we try to be aware about, but sometimes it's very hard to detect. Sometimes our clients aren't even aware—the doctors aren't even aware that they've used an AI tool. It's that lack of transparency in the record, right? So I think those are big issues.
Amori: There's two kinds, apparently, assistive AI and generative AI, and with that subfield of AI that focuses on the interaction between computers and humans through natural language, there's a lot of changes, as Chad mentioned, as everyone has mentioned. So for example, using a large language model for cancer risk in smokers who are Black, the data set used within the AI can't be from a mixed population or for only smokers who are White, otherwise you get the wrong information.
So Irene, briefly, can you tell us what the use of generative AI and how it's problematic for the population that you serve?
Dankwa-Mullan: That's a great question, which we deal with a lot. I mean, basically, one of the most significant challenges with generative AI is the potential for bias in the information it produces. Because we know that they're trained on vast data sets, and it may not be as diverse enough, right, the data sets. And if those data sets, in addition, contains bias, whether it's related to race or socioeconomic status, or even regional healthcare disparities, the biases are amplified, right, in the AI's output.
And I think Chad mentioned it. It's really problematic for patients who are experiencing a social disadvantage, patients who are underserved, who already face barriers to care access issues. And so if a model hasn't been trained on a diverse set of medical records or lack the data representing those communities, its recommendation might be less accurate or less relevant. And so this can lead to treatment plans that overlook these culturally specific health risks or social determinants of health that significantly, we know, impact health outcomes.
Amori: Thank you. So, Danielle, how has the ability to analyze vast amounts of data, leading to breakthroughs in drug therapy and genomics, led to personalized treatment in your practice? I mean, how has it changed your practice? Or has it?
Bitterman: I think first I want to do a little bit of level setting on definition. So assistive versus autonomous AI is not based on the type of AI. It could be generative AI for either, it could be non-generative AI for either. Assistive means it's about the intended use. It means you're using the AI to assist your decision-making. There's a human in the loop. There's a human who's verifying, using the information the AI, but the human makes the final decision. Automated AI is, as it sounds—the AI is making the final decision without so much human oversight. And so obviously, there's differences in safety risk there.
And generative AI, which large language models kind of at the frontier of, is AI that, just as it says, generates a new output, as opposed to just a single or a restricted set of potential options as output. What's interesting with generative AI is it's riskier because it's so open-ended, and it can give so many different types of output, and you therefore can use it in many different ways, so it's harder to risk mitigate. But what's technically interesting is that what it turns out that the training that you need to arrive at a model that is generative, at least a large language model that's generative, also is the training that today has gotten us the best performing AI models. So that's interesting in itself.
So I will say, though, in terms of the breakthroughs of new drug therapies, genomics, that's largely still in the research space. It has not quite percolated into clinic yet. We're still kind of…I think there's huge amounts of potential, but, right now, we are currently standardly using drugs that have been designed by AI, for example.
Amori: Interesting. Chad, but we're showing some literature. There's a bunch of literature out there that shows different things. Some literature says that patients actually prefer an AI to a human doctor, and others say that they like AI, but they want it to be a doctor to be involved. My question that comes to my mind is, as a risk professional, is ultimately, eventually, could physicians be held liable if they don't check AI? What are your thoughts?
Brouillard: Yeah, that's a very interesting question, Geri, and I'm going to borrow one of my old law school professor’s favorite response to every question, which is, it depends, right? But I do think we're in a kind of a double-edged sword scenario. It's interesting because I think the patients in most of those studies push back when they find out that the AI is somehow controlling their care. I don't think patients like that. But when AI is being used as an informational tool, particularly towards patients, they tend to like to get their information from AI. So, it's kind of a weird dichotomy.
But where does this leave the clinician relative to the standard of care? The standard of care is reasonable conduct, right? What an average qualified practitioner would do under similar circumstances. So, it's a double-edged sword because basically if you're using an untested technology, an experimental technology, and it causes harm to patients, that can be considered a ground for liability. You shouldn't have used this new, untested, unvetted…to going to Irene’s example, we used an AI tool, and it had some kind of baked-in bias, and it provided medical advice that could actually harm the patient because of that bias. Well, that would be some potential liability, right?
Now, I mentioned liability is a double-edged sword in this context because also you don't want to be lagging behind your colleagues if you're being reasonable, right? So if all of your colleagues are using a tool, it's been tested, it's been vetted, it's being used widescale, and there's some demonstrated benefit that you can't replicate without AI, then why not use it? Right?
So really, when thinking about liability issues, it essentially boils down to this question: was the doctor being reasonable? And that's how we're going to view it. Was it reasonable to use this AI tool? Did we actually look to see if it was vetted, that there was bias testing? Is there any testing of outcomes? Or was it unreasonable not to use this AI tool, where it's been demonstrated to have better clinical outcomes? That's really what the inquiry would be from a legal point of view.
Amori: Great. Wow. It depends. I knew that was coming, Chad. I knew that was coming. So, we need to bring today's conversation to a close, but before we leave, I want to ask you very quickly to think for a second about the explosion of AI and healthcare, and if you have one point you'd like our listeners to take away, what would that be? And Danielle, I'd like to start with you.
Bitterman: Yeah, it's a great question, very thought-provoking. I think the one point is, as a clinician speaking to other clinicians, this is the time to start engaging meaningfully with the computer science community, putting good faith effort in trying to understand where technology is at now and where it may be going. I think only with being able to get a sense of or trying to predict where we may be in 5 years—given the pace of advances, we're going to be in a very different place 5 years from now—that's going to put us in the best situation to be able to safely use these new methods to the best of their ability. Pretending it's not happening is certainly not the way to set us up for success. So really engaging with the various communities working on AI and trying to learn about it is really going to be important for getting these technologies safe in the clinic and be a safe user of AI in the future.
Amori: Perfect. Yeah, we can't ignore it. We can't ignore it anymore. Irene, what would be the one point you would like our audience to take away? One thing you want them to remember.
Dankwa-Mullan: I think I would say clinicians are concerned about trust, accountability, especially when it comes to integrating generative AI into healthcare. The one thing I want listeners to take away is that while AI has incredible potential, patients want to use it, its effectiveness really hinges on that transparency and that bias management. And so, without clear information on how these models are trained, the data they use, and how they reach their conclusions, we really risk making those decisions based on flawed or incompetent information, especially for vulnerable populations who already face disparities in their care.
Amori: Okay, thank you. So we need to be aware of the data sets we are using and vulnerabilities built in. And Chad, what will be the one thing you want our listeners to take away?
Brouillard: Actually, I think I'm going to double down on what Irene said, in a way. I think the one overall arching theme here is that in order to safely implement AI in the clinical space, we need transparency on many levels, and that means transparency to the patient, that means transparency to the physician so that they understand the tool they're using, its limitations, its potential bias, whether it was vetted or tested, and really the vendor, the software manufacturer, has to be providing those metrics. There's some suggestion in the greater world that the transparency should be publicly accessible. And I think that's probably a good idea in this space because we're all kind of testing out this new technology together, but we're talking about patients’ lives. So, “move fast and break things” is not a great motto in the healthcare space.
Amori: Thank you. And I agree with that. So, this has been an amazing conversation. We've set the stage for moving forward to talk about AI. And I'd like to thank our panelists. You have been incredible. I look forward to speaking with you again. And I'd like to thank our audience for participating today. And I look forward to seeing all of you next time when we discuss another aspect of this healthcare issue from a different Perspective 360.
[music]